Pengchuan Zhang
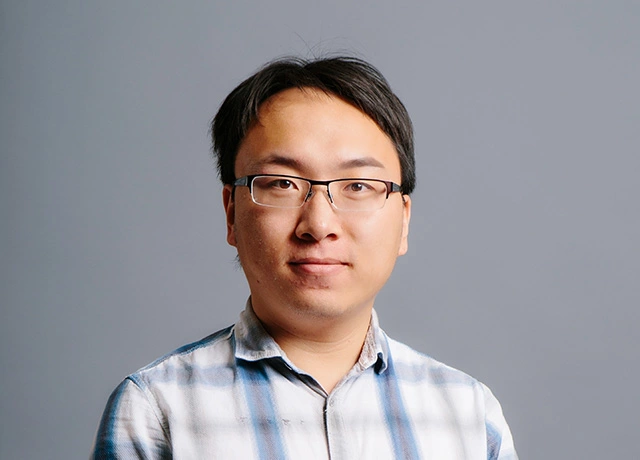
FAIR, AI@Meta
Menlo Park, CA 94025
United States
I’m an AI research scientist at FAIR Computer Vision team of AI@Meta and an affiliate assistant professor in the department of Electrical Engineering, University of Washington. I was a principal researcher at Microsoft Research, Redmond. Before joining Microsoft, I obtained my PhD degree in Applied and Computational Mathematics from Caltech in 2017. My research interests are mainly in the areas of deep learning, computer vision, multimodal intelligence, and theoretical foundations for deep learning.
news
Oct 22, 2022 | Our ECCV2022 workshop “Computer Vision in the Wild” https://computer-vision-in-the-wild.github.io/eccv-2022/ is taking place 9:00am-6:00pm Israeli Time, 11:00pm (October 22)-8:00am Pacific Time, 2:00pm-11:00pm Beijing Time. I will be chairing the monirng section. Welcome to attend the workshop! |
---|---|
Sep 28, 2022 |
We have five papers accepted at NeurIPS2022, all of which are about #computervision and vision-language intelligence. Huge thanks and congratulations to all collaborators!
|
Jun 8, 2022 | 2 pieces of updates on our recent vision-and-language efforts: (i) Our CVPR 2022 tutorial will happen on 06/19/2022; (ii) Our ECCV2022 workshop of Computer Vision in the Wild will happen at ECCV2022 in October 2022. There will be two challenges associated with this workshop: Image Classification in the Wild and Object Detection in the Wild. The challange setup and baselines can be found in our ELEVATER benchmark paper. Stay tuned for more details. |
May 3, 2022 | I’m starting a new position as Research Scientist at Meta AI for VR. I will continue my long-term pursuit of CV and Multi-modal intelligence at my new position. Looking forward to work with colleagues and the entire community, to build intelligent and trust-able technologies for the metaverse, and to push the research frontier of deep learning, CV and multi-modal. |
Mar 11, 2022 | Gave a talk at Applied and Computational Mathematics Seminar at University of Wisconsin at Madison. The talk is about my ICLM 2021 work “Multiscale Invertible Generative Networks for High-Dimensional Bayesian Inference”. The talk slides can be found at here. |
selected publications
-
Using statistics to automate stochastic optimizationAdvances in Neural Information Processing Systems 2019
-
Vinvl: Revisiting visual representations in vision-language modelsIn Proceedings of the IEEE/CVF Conference on Computer Vision and Pattern Recognition 2021
-
A convex relaxation barrier to tight robustness verification of neural networksarXiv preprint arXiv:1902.08722 2019
-
Attngan: Fine-grained text to image generation with attentional generative adversarial networksIn Proceedings of the IEEE conference on computer vision and pattern recognition 2018
-
Provably robust deep learning via adversarially trained smoothed classifiersarXiv preprint arXiv:1906.04584 2019
-
Multi-scale vision longformer: A new vision transformer for high-resolution image encodingarXiv preprint arXiv:2103.15358 2021
-
Multiscale Invertible Generative Networks for High-Dimensional Bayesian InferencearXiv preprint arXiv:2105.05489 2021
-
Florence: A New Foundation Model for Computer VisionarXiv preprint arXiv:2111.11432 2021
-
Grounded Language-Image Pre-trainingarXiv preprint arXiv:2112.03857 2021